Q&A: AI
Kickstarting AI and smart data in resource-limited health systems
Low and middle-income countries have the most to gain from the public health benefits of AI and digital tech, but also the most to lose. That’s the conclusion of a report led by the Novartis Foundation and Microsoft, which sets a roadmap for AI maturity in global healthcare. Chris Lo speaks to Novartis Foundation head Ann Aerts about how best to accelerate the AI revolution in resource-limited settings.
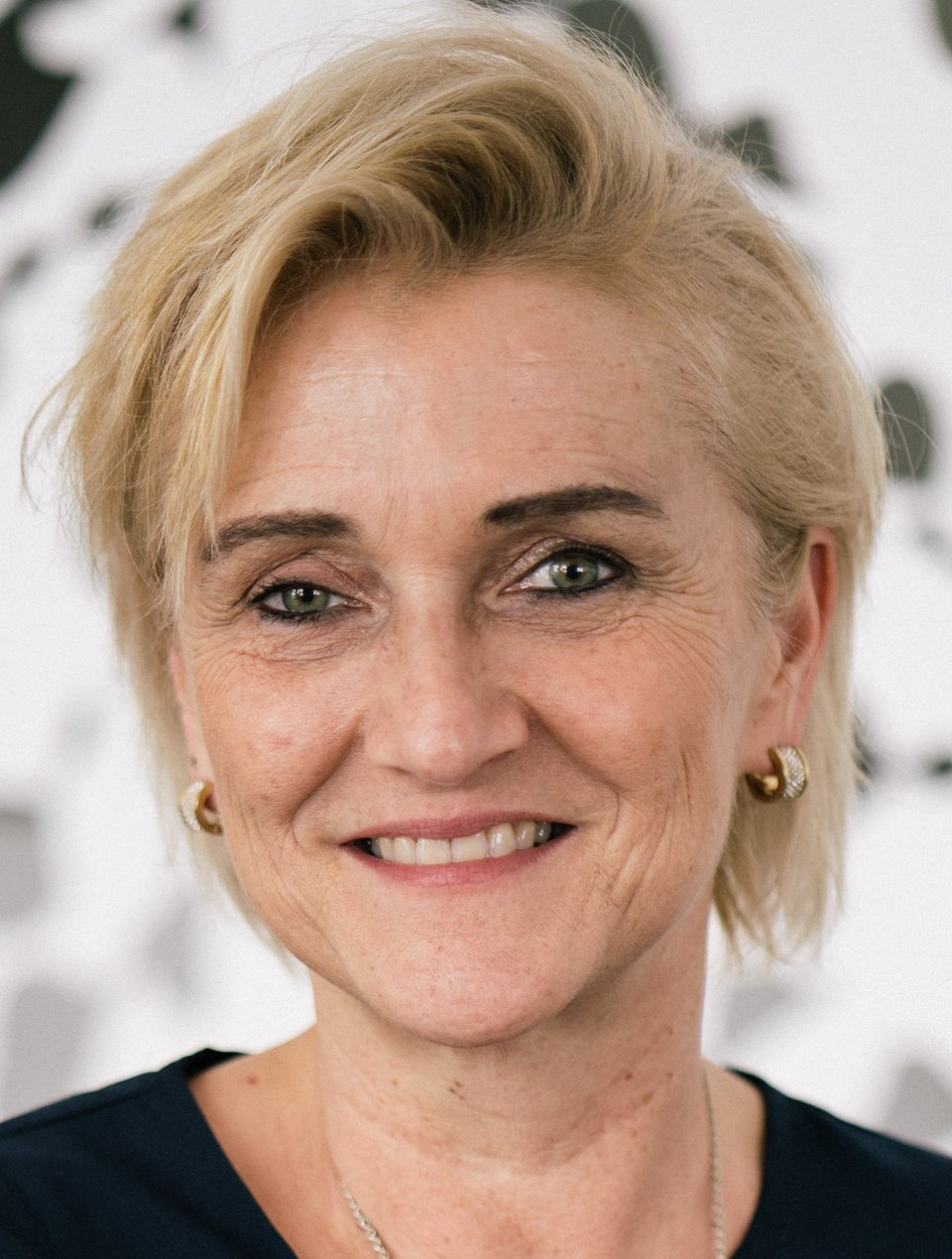
I
n September, the Novartis Foundation – the philanthropic, non-profit arm of the Swiss pharma giant – partnered with Microsoft to publish a new report entitled ‘Reimagining Global Health through Artificial Intelligence: the Roadmap to AI maturity’. The report constitutes a comprehensive landscape analysis of the evolving role of artificial intelligence (AI) and digital technologies in global healthcare, and is the result of extensive consultation and research by the Broadband Commission for Sustainable Development’s digital health working group.
Above all, the report provides a roadmap for maturing and tapping into the incredible potential of AI and big data as a driver of global health, particularly in low and middle-income countries (LMICs). The team’s research spanned the globe and found that, just as in the wealthiest economies, LMICs are already taking advantage of AI and smart data innovations to improve health at the clinical and population level.
The report picks out particularly striking examples of this, including Babylon Health’s project in Rwanda – Babyl Health – which offers AI-powered digital consultation and remote triage services to thousands of citizens every week. In India, meanwhile, private healthcare company Apollo Hospitals has been working with Microsoft’s AI Network for Healthcare, deploying AI to develop a country-specific cardiac risk model that has been applied to more than 200,000 people so far.
LMICs often bear the brunt of broad healthcare challenges such as rapid urbanisation, shortages of skilled health workers and rising levels of both chronic and infection disease. As such, these nations have the potential to gain the most from accelerating the deployment of AI and smart data, but also the most to lose if they miss out on the opportunity. The ongoing Covid-19 pandemic serves as a potent reminder that emerging public health threats will be best tackled by healthcare systems that are further along in the transition towards predictive medical models, telemedicine and more.
Here, Novartis Foundation head and co-chair of the Broadband Commission’s digital health working group Dr Ann Aerts discusses the potential of AI and digital tech in resource-limited settings, and how best to accelerate the safe and fair deployment of these life-saving innovations.
Chris Lo:
What makes AI and data science so important for healthcare in low-income countries?
Dr Ann Aerts:
I think it’s a fantastic opportunity. We live in a digital era, where we can start reimagining the way we deliver health and care around the world, because that is really needed.
If you look at all the challenges that health systems in low- and middle-income settings have to face, with the dual burden of disease [rising rates of both noncommunicable and infectious diseases], rapid urbanisation, shortages of skilled health professionals – with all of that together, along with many other things, it’s pretty much impossible for health systems that are under-resourced and insufficiently prepared to address all these challenges.
So we need to redesign and re-engineer the way we deliver health and care, and what is a better opportunity than using data, digital and AI to do so?
To what extent do you think the ongoing coronavirus pandemic has accelerated the development and deployment of AI and smart data technologies in healthcare?
I think we’ve been catapulted into the digital era, and the advances we’ve made in the few months since the beginning of the pandemic have been equal to years previously. If you think about how telemedicine has been struggling to get reimbursed, even the very mature models of providing virtual care by telephone or by WhatsApp have been struggling to get financed, to get reimbursed.
And it was taken up immediately, because we see that those countries that had that mature model already operating had a much bigger advance in how to handle the pandemic than others did. Even the most sceptical health professionals who never wanted to touch digital for their health provision have been carried over the line, and are now convinced about it.
The doctor-to-population ratio tends to be particularly low in LMICs. Will AI and big data become a key enabler for doctors and other healthcare professionals to better manage large patient populations?
Absolutely. You know, I’m a medical doctor myself and in the first part of my career I worked in low and middle-income settings, mainly as an emergency physician in conflict areas.
I was dropped by plane in the middle of an area, and I rode my bicycle from village to village to see the patients that the village health workers had identified for treatment. You cannot imagine that you would have to do that now – you can do that by phone, you can coach less-skilled health workers in patient care by phone or WhatsApp.
The reach you can achieve using virtual care and AI is absolutely superior to in an in-person health system, because you can centralise your expertise. We did that in our telemedicine programme in Ghana, which has become the national telemedicine service in that country.
We really looked at the needs of the system – they have a lot of people living in quite remote areas where there’s absolutely no access to a doctor or specialist. What we established were simple call centres where village health workers can call into the staff at the reference hospitals, who are the experts and really experienced midwives or doctors or surgeons.
They are coaching less-skilled health workers on the ground. With that, you reduce unnecessary referrals and solve the problem for 70% [of patients].
How can AI and data science help reduce health inequalities in LMICs, and what is the best way to roll out these innovations in the most equitable way?
The digital divide in the world has become the new face of inequality. So we have to make sure that we roll out and integrate these solutions properly. To do so, you have to think about the people who will use it, the people who need it. If you come with a perfect solution that is well-intended but doesn’t correspond to an existing need, it will never get to scale.
You will face a lot of resistance from the health professionals, and again that creates inequity, or a fragmented landscape such as we saw a lot with the digital solutions around the world, which were introduced by well-intentioned donors, but you ended up with systems where you have a nurse on the ground with seven mobile phones – one for TB, one for HIV and so on.
Is Apollo Hospitals’ cardiac risk AI model in India a template that other countries might be able to follow on the way to more preventative healthcare models?
Potentially, but I think the solutions can be developed in one country with a set of data that is representative for the population in that country, as Apollo did.
They looked at millions of data points on cardiovascular disease that they have in their hospitals, because they knew that the currently available risk scores that we use around the world are based on Caucasian male data, so from the good old Framingham studies from 70 years ago, and they’re not applicable to populations around the world. Something very important for the data and the tools that come out of those assets, is that they are representative of the populations they will have to be used in.
So you can definitely develop a tool like that in a population data-set from India, but then validate it on another population data-set. That’s exactly what we’re doing, for example, in Brazil, by localising those kinds of solutions with the data we have in situ there. We don’t have to transport the data – you can test it in another setting and see if it’s outperforming the existing risk scores. That is the beauty of AI; you can constantly improve your model.
And from governments’ perspective, what are the most important things to consider when LMICs are working on how to deploy AI fairly and safely?
Could guidance from major regulators and multilateral groups like the WHO play an important role here?
Creating the governance and regulatory systems that keep people safe, and make people trust those solutions and the models– that’s the role of governments and institutions such as WHO [World Health Organization] and others, to set those standards.
Everybody says you have to set up your regulatory system, but we can look at others, how they did it – like FDA now launching its first centre of excellence for digital solutions – let’s look at those and see how we can help countries to learn from those insights, to do it better themselves.
It’s not a simple thing to set up a regulatory system, and definitely not for AI solutions in health. Because every regulator looks at static solutions in general, and the AI model can never be static, because the moment you’re evaluating it, it’s already changed two days later because it’s improving itself.
This needs a whole other system of regulation – that’s why we will have to look at what Europe is doing, what the US is doing. In that sense, we can learn from those regulators. Europe is quite strong in its privacy laws and the GDPR [General Data Protection Regulation], so that’s something other countries are looking at as well.
Main image: Novartis Foundation head Dr Ann Aerts. Credit: Novartis Foundation