Innovation
Predicting cardiac risk: new approaches in AI and blood testing
Common methods used to predict cardiac risk can be frustratingly inaccurate, but innovations in both blood testing and machine learning technology could change that. From chemical to digital, Abi Millar profiles these two very different research strands that are working to boost the prevention of deadly cardiac disease.
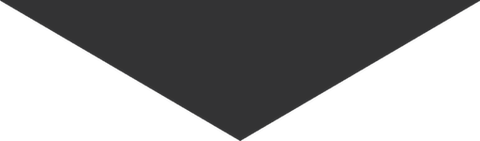
H
eart disease is extremely common. In the UK, around 7.4 million people live with the condition, and someone is admitted to the hospital with a heart attack every five minutes. Globally, cardiovascular disease (mostly heart attacks and strokes) remains the leading cause of mortality.
Unfortunately, predicting heart disease remains an inexact science. Doctors mostly rely on assessments of cholesterol, blood pressure, lifestyle factors and health conditions to determine who might be susceptible.
As a result, this remains a fertile area for research. If we can home in on the people most likely to suffer heart attacks, years before they actually do so, we can stand a better chance of accurately targeting interventions.
“Even though there have been significant gains in the last decade in terms of diagnosing heart disease and treating heart attacks, our predictive capability for what’s going to happen with these patients in the long term has never been so good,” says Dr Luis Eduardo Juárez-Orozco, of the Turku PET Centre in Finland. “Predicting specific events on an individual level has always been a challenge for cardiovascular researchers.”
Artificial intelligence to the rescue
Juárez-Orozco’s own research points to an intriguing solution to the problem. With a background in artificial intelligence, he has developed a machine-learning algorithm that can predict death or heart attack with greater accuracy than humans.
The study looked into the medical records for 950 patients with chest pain. On top of their clinical data (ten variables in total, including sex, age, smoking and diabetes), all had received a coronary computed tomography angiography (CCTA) scan, which produced 58 pieces of data. Some patients also had a PET scan, which yielded 17 pieces of data.
By the end of the follow-up period (six years on average), there had been 24 heart attacks and 49 deaths from all causes.
I would estimate we’re getting a 10% improvement on what we were able to do before.
Juárez-Orozco fed this data into an algorithm called LogitBoost. Through a process of iteration, the algorithm was able to develop a predictive model for which patients had a heart attack or died. While not perfect, this model was a step beyond the ‘risk scores’ typically used by doctors.
“The metric we use is the ‘area under the curve’ (AUC), which is a measure of how well the model is able to distinguish people who have the event from people who don’t have the event,” says Juárez-Orozco. “With traditional risk scores, the AUC is clearly suboptimal – we’re talking in the order of 0.65 or 0.70. We were able to go to 0.75 or 0.80, so I would estimate we’re getting a 10% improvement on what we were able to do before.”
He points out that, while a 10% improvement might not sound too impressive, in practice it could translate into real clinical benefits.
“In other industries, there have been a lot of efforts to incorporate machine learning,” he says. “For instance, Netflix were able to lift their predictions for movie recommendations by about 10%, and that boosted their capability as a company massively. That’s in the order of what we’d like to see for improvement.”
The future prospects
While machine learning is already a staple of day-to-day life (think Google’s search algorithm or facial recognition at airports), healthcare is lagging behind in this regard.
“Medical applications will always have to be way more regulated than industrial applications, simply because of the potential consequences for patients and clinicians if something goes wrong,” explains Juárez-Orozco. “We don’t know yet where the responsibility has to be placed – does it fall to the clinician who makes the decision or the developers who make the model, or does it fall within the normal rate of error? That’s why it takes a little more time for these kinds of technologies to permeate into healthcare and that’s why we have to pay a lot of attention to designing them properly.”
He feels that, rather than displacing a clinician’s insights, systems of this might ultimately be used as a kind of support system that would make their life easier and lighten their workload.
Medical applications will always have to be way more regulated than industrial applications.
“These systems are already remarkably good at ruling out cases with diseases, so maybe within the next few years we can start leaving that to the systems so the clinicians can focus on more complex cases,” he says. “That would really improve the quality of care and the utilisation of time.”
For now, his team are collaborating with the University Medical Centre in Groningen in the Netherlands, with a view to refining their system further.
“It is definitely within the scope of our approach to expand these collaborations to anywhere data can be found and anyone who’s interested in these topics,” he says. “Several heads work better than one.”
A chemical alternative
Across the Atlantic, a very different approach to predicting cardiac risk is being trialed. According to a study published in the journal Circulation, Abbott’s High Sensitive Troponin-I blood test could predict the chance of developing a cardiac event years before any symptoms emerge.
Troponin blood tests are already widely used to diagnose heart attacks. When a patient has suffered a heart attack, troponin (a group of proteins found in the cardiac muscle) is released into the blood in large quantities. There is a strong correlation between the amount of troponin and the amount of damage sustained.
If a patient hasn’t actually had a heart attack, their troponin levels would previously have been considered undetectably low. However, the new test is sensitive enough to detect elevated levels of troponin in healthy adults.
The new test is sensitive enough to detect elevated levels of troponin in healthy adults.
Using data from the ARIC study – an epidemiologic study on the causes of atherosclerosis – researchers looked at more than 8,000 adults aged 54-73, whose blood was drawn in 1998. While none of the participants had been diagnosed with heart disease at the time, 85% had detectable troponin.
Compared to participants with low levels of troponin-I, those with higher levels were more than twice as likely to have had a cardiac disease event by 2013. They were nearly three times more likely to have had a stroke, and more than four times more likely to be hospitalised with heart failure.
Dr Christie Ballantyne, professor of medicine at Baylor College of Medicine in Texas and the corresponding author of the study, believes this test should be added to routine physical assessments in middle-aged and older adults.
“It’s interesting, there’s been a lot of talk about the measurement of a high sensitivity assay for C-reactivity protein as a marker of inflammation, but Tropnonin I was a better test than hsCRP, which is the one that’s used very commonly clinically,” he says. “While there needs to be more research, it could be very beneficial, as heart failure hospitalisations are a big problem for society.”
While a blood tests and an algorithm are two very different strategies, both could play an important role in tomorrow’s risk assessments. In years to come, simply taking someone’s cholesterol and blood pressure measurements may come to seem like an unsophisticated approach.