Data management
Gaining value from data compliance with Master Data Management 2.0
Unless life sciences firms can exploit their product data compliance efforts to transform everyday operational processes, they will limit the return on their investment as they prepare for new requirements. AMPLEXOR’s Siniša Belina explains how a new approach to managing data could help.
The latest regulatory requirements affecting life sciences – including ISO IDMP and new FDA Standardization of PQ/CMC data elements and terminologies – are so demanding that it is prompting many companies to completely rethink the way they use product information. And this promises to serve them well, as long as they automate more of what they do with these data assets.
Up to now, we have advised companies to work towards a ‘master data’ scenario – a single, central source of product truth which can inform numerous use cases. The next stage is to exploit this valuable repository to automate and streamline processes that currently take an inordinate amount of time – from regulatory submissions preparation to global product labelling and patient information production.
We call this extended approach to product data exploitation Master Data Management 2.0 – MDM 2.0 for short.
Creating repeatable value by using master product data
The impact of using master product data to automate content preparation is potentially significant. Currently, companies create regulatory submission documents, fill in forms, generate labels, packaging and patient information more or less from scratch each time there is a new requirement.
This involves having to call up different systems, and look through various tables and spreadsheets to find data to manually copy and paste into the new output. It’s is a hugely laborious process which is fraught with risk of getting some detail wrong, using out of date information, or failing to conform to a market’s particular requirements.
But with easy, confident access to the correct content components, organisations could be populating new documents at the touch of a button – automating at least 90% of the process of content generation, so that all that remains is for someone to add any finishing touches and check everything over.
If existing content exists primarily in monolithic form, in previous documents for instance, it is of little value for future use.
Automated content creation relies on two things: good, definitive master data and the ability to pull in and mix and match approved data components according to the given context. If existing content exists primarily in monolithic form, in previous documents for instance, it is of little value for future use – unless someone checks and re-enters the information each time.
If the latest version of that content exists in more granular form, in a central data bank – as a series of searchable and easily extractable content assets – not only is it easy to repurpose again and again, but this core content only has to be updated or amended once, in one place. Those edits can then be applied across all new use cases, with a few simple clicks. Crucially, everything can be viewed and monitored in one place, too.
This is the kind of process that happens as standard in other markets where there is a lot of live content to keep track of across sprawling operations. And, at last, proof-of-concept projects are beginning to take shape in life sciences. Here, companies are starting to create templates for common document creation, based on master data.
In this kind of ‘structured authoring’ scenario, output is generated with minimal effort. Once the context has been indicated (the product, the type of content needed), the correct data assets can be automatically pulled together to form the target content.
Collapsing content production cycles
For standard application forms, where no customised tweaking is required, 100% of the document compilation could be automated, accurately matched to the given market and target language.
The expectation is that the time savings will be at least ten-fold: so where new content preparation has previously taken 50 man days, it will now take just five. These are phenomenal efficiency gains, offering to significantly accelerate companies’ speed to market while freeing up experts to focus their time more on higher purposes.
For life sciences organisations that still rely on very manual, decentralised processes for putting together product-related content, the transformation presented by MDM is huge.
Assuming the chosen content management system is able to take care of document creation and approved local translations simultaneously, there should be no need to create each local version of documents separately. Structured content templates will be able to pull in the correct, pre-verified text fragments in each language, meaning there is no need to re-translate content each time. That’s because approved translations of existing wording and text extracts already exist in the master database.
For the majority of life sciences organisations that still rely on very manual, decentralised processes for putting together product-related content, the transformation presented by master data management (MDM) and its next-generation manifestation, MDM 2.0, is huge. On top of the time and efficiency gains, it offers company HQ much greater confidence and oversight of the content being put out across global operations – minimising the risk of product recalls resulting from inaccurate or incomplete information being submitted, or the wrong phrasing being used.
Artificial intelligence: the next level of process automation
The vision for MDM 2.0 isn’t confined to structured authoring of content. It’s about boosting what companies can do with data to improve their operations and business impact.
While initial projects might focus on internal operational data about their own products and processes, there is great scope to enhance this with external intelligence – for instance, data about market conditions, or evolving regulatory requirements in different regions and countries. The more complete and rounded the data that is input into central systems, the easier it becomes to plan for and manage new requirements – and improve success rates.
There is much to be excited about, particularly as artificial intelligence and machine learning enter the picture, helping systems to ‘learn’ how to produce better output, or the conditions most likely to result in a new marketing submission being accepted first time.
Smart algorithms could learn to recognise and adapt to common edits that users are making to complete or finesse given document output.
As companies move towards automatically generated documents, smart algorithms could learn to recognise and adapt to common edits that users are making to complete or finesse given document output. Instead of admin staff conducting periodic reviews and restructuring the templates accordingly, an AI-enabled system would anticipate and propose improvements based on frequent changes that users have had to make.
When building a submission, the system might suggest which documents to include; which contributors to involve in the authoring/review/approval process; how to set up the ti
melines – perhaps even anticipating questions that are likely to come from the authorities based on points raised previously for related or similar submissions. The scope is probably much bigger than we’re even able to imagine at this early stage.The vision they must work towards is one in which teams will simply tell a system what type of documents they need… leaving the technology to do the rest.
In the meantime, the goal should be to automate all of the routine activities that take away time users could be allocating to other, more demanding tasks. The enabler for this is the creation of a comprehensive master data model – one that also includes active relationships and dependencies between the data, in a way that can drive new efficiencies and increased impact through proactive process automation.
The vision they must work towards, and which is encapsulated by MDM 2.0, is one in which teams will simply tell a system what type of documents they need, for which product, and for what purpose (country/region, type of submission, and so on), leaving the technology to do the rest. That could be generating new documents from the master data and appropriate structured templates, or directing users to existing documents and even proposing updates, corrections, improvements based on previous use of the system or newly entered data, for example about the latest regulatory requirements).
As the industry starts its IDMP preparation in earnest, what better time to redraw the parameters to ensure that any changes not only cover their costs but pay for themselves many times over.
About the author
Siniša Belina is senior life sciences consultant at AMPLEXOR Life Sciences. He started his professional career at Pliva (now a member of the TEVA Group), where in addition to his responsibilities in manufacturing, he also engaged in successful EDMS implementation projects.
Belina later joined KRKA’s Regulatory Affairs Department, and finally moved to AMPLEXOR. He applies his detailed knowledge of pharmaceutical documentation and processes to areas of business process analysis and EDMS optimisation.
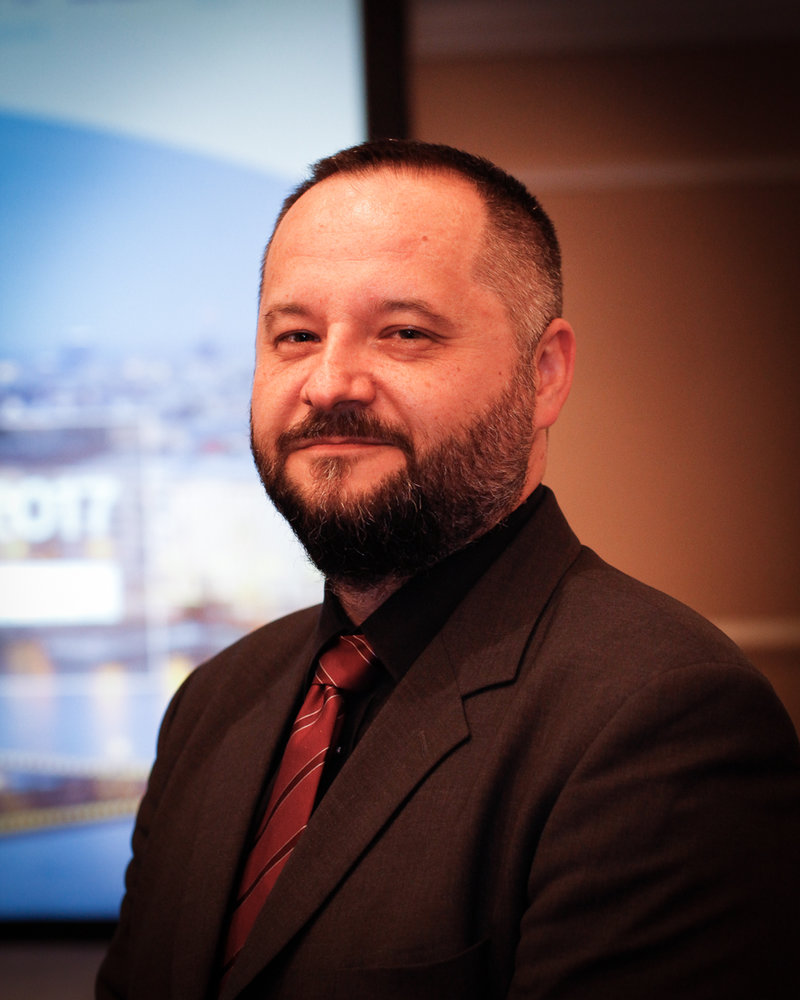