Feature
The rise of generative AI in diagnostics
Generative AI models are transforming IVD technologies, offering various applications and benefits for digital pathology, colour normalisation and smart diagnostics. By Bernard Banga.

Credit: Shutterstock/Deemerwha Studio
Digital pathology (DP) has transformed the traditional practice of analysing tissue under a microscope into a computer-based workflow. DP uses slides composed of cells or tissues that are digitised using a high-resolution scanner in a process known as whole-slide imaging (WSI).
'Recently, task-specific artificial intelligence (AI) (e.g. convolutional neural networks (CNN)) has risen to the forefront achieving above-human performance in many image-processing and computer vision tasks,’ observed a recent study published in Laboratory Investigation by a group of authors led by Asim Waqas, graduate research fellow in the Department of Machine Learning at the H. Lee Moffitt Cancer Center and Research Institute, Tampa, Florida, USA. AI-driven analysis tools can automate various tasks, including recognising objects and images, understanding and generating language text, and making predictions based on historical data. However, the performance of task-specific AI/machine learning (ML) models depends on the availability of well-annotated training datasets, which is a limiting factor for AI/ML development in pathology.
Generative AI models: an expert companion tool for pathologists
Today, AI models face challenges in generalising to new datasets or coping with unseen variations in image acquisition, staining techniques or tissue types. The current surge of foundation models and generative AI processes vast amounts of medical data. These models ‘provide in-context learning, can self-correct their mistakes, and promptly adjust to user feedback,’ explained Waqas, co-author Ghulam Rasool and colleagues. New pathology-specific generative AI tools, based on various multimodal foundation models encompassing images, text and tabular data, are poised to serve as expert companions for pathologists. ‘These multimodal foundation models utilize diverse data sources for improved generalization and adaptability,’ observed the authors. In 2024, generative AI models are beginning to flourish in the field of automated histopathology, particularly in cancer diagnosis and grading. For instance, Paige Inc., based in New York, USA, has developed an AI tool to help pathologists detect, grade and quantify prostate cancer. Paige Prostate has obtained CE marking and FDA approval as a Class II medical device. In early January 2024, Paige announced the release of a groundbreaking multi-tissue detection model with the ability to identify cancer across more than 17 different tissue types, including skin, lung, and the gastrointestinal tract, along with multiple rare tumour types and metastatic deposits.
Generative AI does, however, come with associated challenges and limitations: "Further research and development efforts are needed to fully realize the current AI wave’s potential to ensure their safe and effective implementation in clinical practice," observed Waqas and colleagues.
GAN: a novel paradigm for colour normalisation
In medical imaging applications, colour is typically handled with little standardisation. The purpose of colour normalisation is therefore to generate colour image data with an identical or similar perceptual response when evaluated by human operators. Several colour normalisation algorithms have been proposed in the literature for digital pathology and dermatology, specifically addressing issues with colour and intensity artifacts. Generative adversarial networks (GANs) are the latest emerging framework for generating synthetic images and time-series data. "In this generative model, a network known as the generator is trained by attempting to trick a second model, known as the discriminator," explained Massimo Salvi, assistant professor in the Department of Electronics and Telecommunications at Politecnico di Torino, Turin, Italy. GANs can generate synthetic datasets to overcome the fact that datasets stained at different institutions often exhibit a great deal of variation in colour and intensity. Acquiring high-quality labelled training data can be expensive and time-consuming. Generative models ‘can also be used to virtually stain tissue, reducing the tissue preparation overhead’, explained a group of authors led by David Morrison, a lecturer at the School of Medicine, University of St. Andrews, Scotland, in a study published in The American Journal of Pathology.
GAN-based approaches are particularly useful for normalising stains in histopathological images. Three different methods are used for colour normalisation: Pic2Pix GANs, which facilitate image-to-image translation; stain-style transfer networks, which are adept at transferring histopathological staining styles while preserving tissue morphology; and cycle GANs, which are highly effective for tasks such as style transfer and image-to-image translation. However, ongoing research is essential in the rapidly developing field of generative AI-based tools. Addressing four critical challenges – maintaining robustness to image variations and modalities, preserving crucial clinical features during image translation, efficiently managing unpaired data for translation tasks, and ensuring the clinical relevance and reliability of translated images for accurate diagnoses – will further enhance the capabilities of these tools.
Combining generative AI with biosensors and lab-on-a-chip technology
The latest trend is smart diagnostics driven by integrated biosensors, cloud computing and generative AI models. This emerging point-of-care technology encompasses several key elements, as described in a paper published in Sensors by a team led by John T. McDevitt, professor in the Department of Molecular Pathobiology, Division of Biomaterials at New York University (NYU) College of Dentistry. These include a universal instrumentation platform able to facilitate a multitude of diagnostic tests, highly scalable biosensors supported by microfluidics for assay processing, analysis software for digitising chemistry or biology, generative AI inference and clinical decision support, and intuitive reporting and integration with electronic health records.
McDevitt’s team has developed a smart diagnostics platform for biochemical analyses that uses a compact instrument to process programmable assay cartridges (microfluidic devices pre-populated with liquid and dried reagents). Image analysis ‘digitises biology’ by converting fluorescence signals into features that can be read by machine learning algorithms to infer disease and health outcomes, producing an intuitive ‘score’ reported to patients and providers.
This multiclass, multiplex measurement system has been validated through a series of large clinical studies, and ‘has been applied to predict outcomes in oral, ovarian, and prostate cancers, as well as cardiovascular disease, trauma, drug abuse, and COVID-19 severity,’ stated the team in their paper.
This point-of-care compatible platform can perform rapid immunoassays in around 10 minutes for drugs, 16 minutes for a COVID-19 severity panel, and 20-25 minutes for oral cytology, and the system completely automates the sample and reagent handling steps and thus requires minimal training to operate.
The utility of the platform may further extend to multiple cell-based oncology tests using cross-reactive biomarkers spanning oral, colorectal, lung, bladder, oesophageal, and cervical cancers, and it is well-positioned to improve patient care, management, and outcomes through deployment of this resilient and scalable technology, stated McDevitt.
AI-based digital pathology: the Korean experience
South Korea is championing several AI data platform projects in diagnostics, including a collaborative open digital pathology artificial intelligence platform, Deep Uro, and Data Dam. "Digital pathology is evolving in our country as a platform for whole-slide imaging, telepathology, digital image analysis, computer-assisted diagnosis, and consulting in multidisciplinary meetings," explained Yosep Chong, associate professor in the Department of Hospital Pathology at the Catholic University of Korea.
The Catholic Medical Center is part of South Korea’s biggest university, and operates a chain of 8 branches with 6500 beds, 2800 doctors and 50 pathologists, covering 50% of the Korean population area. The country has 216 pathology labs, which are spread across 85 general hospitals, 82 university hospitals, and 49 commercial laboratories. Among these labs, 13% utilise 63 digital pathology systems, primarily supplied by Leica Microsystems GmbH, 3D Histech Ltd., Hoffmann-La Roche AG, Koninklijke Philips N.V., and Hamamatsu Photonics K.K.
"The implementation of digital pathology and AI applications is gradually expanding over time," noted Chong, observing that between 2020 and 2023, there were 9 FDA-approved and CE-marked pathology AI models. Generative AI could address four major obstacles to implementing digital pathology: their pricey systems, increased turnaround time to deliver a pathology report after a specimen has been collected and sent to the laboratory, the additional personnel for scanning and quality check, and the adaptation curve, concluded Chong.
The paper showcased attempts to make GPT-4 produce data that supported an unscientific conclusion – in this case, that penetrating keratoplasty had worse patient outcomes than deep anterior lamellar keratoplasty for sufferers of keratoconus, a condition that causes the cornea to thin which can impair vision. Once the desired values were given, the LLM dutifully compiled a database that to an untrained eye would appear perfectly plausible.
Taloni explained that, while the data would fall apart under statistical scrutiny, it didn’t even push the limits of what Chat-GPT can do. “We made a simple prompt […] The reality is that if someone was to create a fake data set, it is unlikely that they would use just one prompt. [If] they find an issue with the data set, they could fix it with consecutive prompts and that is a real problem.
“There is this sort of tug of war between those who will inevitably try to generate fake data and all of our defensive mechanisms, including statistical tests and possibly software trained by AI.”
The issue will only worsen as the technology becomes more widely adopted too. Indeed, a recent GlobalData survey found that while only 16.1% of respondents from its Hospital Management industry website reported that they were actively using the technology, a further 26.8% said either that they had plans to use it or were exploring its potential use.
Nature worked with two researchers, Jack Wilkinson and Zewen Lu, to examine the dataset using techniques that would commonly be used to screen for authenticity. They found a number of errors including a mismatch of names and sexes of ‘patients’ and lack of a link between pre- and post-operative vision capacity.
In light of this, Wilkinson, senior lecturer in Biostatistics at the University of Manchester, explained in an interview with Medical Device Network that he was less concerned by AI’s potential to increase fraud.
“I started asking people to generate datasets using GPT and having a look at them to see if they could pass my checks,” he said. “So far, every one I’ve looked at has been pretty poor. To be honest [they] would fall down under even modest scrutiny.”
He acknowledged fears like those raised by Dr. Taloni about future improvements in AI-generated datasets but ultimately noted that most data fraud is currently done by “low-skill fabricators,” and that “if those people don’t have that knowledge, they don’t know how to prompt Chat-GPT to have it either.”
The problem for Wilkinson is how widespread falsification already is, even without generative AI.

Caption: The US Pentagon is seeking to reduce carbon emissions through a range of programmes, but will it go far enough? Credit: US DoD
The mine’s concentrator can produce around 240,000 tonnes of ore, including around 26,500 tonnes of rare earth oxides.
Gavin John Lockyer, CEO of Arafura Resources
Total annual production
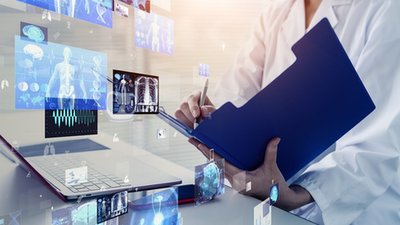
$345m: Lynas Rare Earth's planned investment into Mount Weld.

Caption. Credit:

Phillip Day. Credit: Scotgold Resources