Feature
AI is reimagining imaging, but how far can we go today?
Artificial intelligence (AI) is already having a huge impact on radiology departments. However, the onset of advanced analytics and machine learning may be tempered by systemic and economic barriers. By Jo Shorthouse.
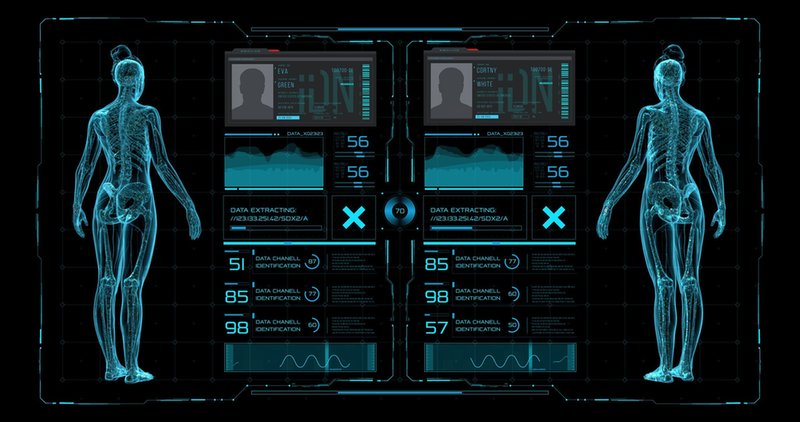
Credit: GettyImages
On average, a radiologist looks at 50,000 images in a 12-hour shift. To put this into perspective, 50 years ago a radiologist would have looked at around 500 images per shift.
In 2010, it took three and a half years for medical data to double - only 10 years later in 2020, it took 73 days. There are close to 6000 medical journals published every year, putting out close to a million articles a year.
This data includes in vivo data from machines such as CT, MRI, and ultrasound, in vitro data or omics data coming from the laboratory, pathology departments, electronic health records and increasingly from wearables.
“There is an avalanche of data, and no one can deal with it,” says Mathias Goyen, GE Healthcare’s chief medical officer for EMEA, and a radiologist by profession. “The amount of data in healthcare is mindblowing."
Mindblowing it may be, but also overwhelming for those working with imaging every day. The use of artificial intelligence (AI), however, can help with this data tsunami by spotting patterns that help physicians find the cause of diseases before it’s too late.
This is the point of using AI in imaging, Goyen stresses to Medical Device Network - to provide the clinician with accurate data to form a fast, reliable diagnosis so that the patient has more treatment options than they do today.
AI, machine learning and advanced analytics are impacting radiology today at the individual level by embedding AI capabilities into scanners, ultrasound, and x-ray machines, and at the departmental level to streamline workflows and remove waste from the system in the radiology department.
The American College of Radiology’s (ACR) Data Science Institute found that in 2020 around 30% of radiologists were using AI as part of their practice. Of those using AI in clinical practice, most were applying it to enhance interpretation, most commonly detection of intracranial hemorrhage, pulmonary emboli, and mammographic abnormalities.
What is stopping us?
While the onset of AI is a very real proposal for many radiology departments, barriers to access remain. The most important of these is the access to high quality data, said Goyen. “You put garbage in, you get garbage out,” he tells Medical Technology. GE Healthcare is frequently offered data from hospitals globally, but on closer inspection it does not meet its quality standards. “There are real challenges in getting accurate and effective data of high-quality that have proper labelling and annotation useful for training,” says Goyen.
Integrating AI into data workflows can stop radiologists from fully embracing the potential of the technology. “If it gets too complicated, it's a huge barrier for adoption so it must be seamlessly integrated. If the doctor doesn't even know that AI is at work, we at GE Healthcare, have done everything right,” Goyen explains.
GE Healthcare’s imaging figures are impressive; 259,000 patients supported daily using GE imaging technology, with two billion patient scans managed by GE technology per year. There are four million GE Healthcare imaging, mobile, diagnostic, and monitoring devices worldwide.
The company’s March 2023 acquisition of Caption Health to support GE Healthcare’s $3bn ultrasound business augments this position in the market, and builds on the 2021 purchase of BK Medical for $1.45bn that incorporates the relatively new field of real-time surgical visualisation to the company’s pre- and post-operative ultrasound capabilities.
The Caption Health buy is a “perfect fit” according to Goyen, explaining that Caption’s AI solutions will be primarily integrated into the point of care and handheld business. Acquisitions such as this one help to bring AI into the world of imaging without radiologists or technicians having to worry about integrating advanced data analytics into workflows as an add-on.
The human touch
Where there is data there is ethics. It is easy to become swept up in the potential of AI, yet one must not forget the questions from patients. Who is responsible if an AI algorithm makes an incorrect diagnosis? Can the patient sue the hospital? Can the doctor then sue GE Healthcare because the AI is from that company? And how can we ensure that patient privacy and confidentiality when using AI in healthcare is respected? These are just some of the questions that are largely still to be answered. While Goyen calls this “a low barrier” to the widespread adoption of AI, it is one that remains and will need continual consideration.
"It's a change management process. It takes some time, you need active ambassadors who are willing to implement this, who really burn for the idea,” says Goyen when asked about resistance to change within the radiology clinic.
“You can either be an early adapter and have the chance to shape and influence its role and impact in radiology, or you do watchful waiting, but if you do that you can only ever be a follower.
While the enthusiasm for AI within imaging is strong, the barriers to implementation and adoption should not be underestimated. Those using AI within their clinic are settling into a huge change in their working life. And let us not forget the patient, healthcare is based on trust. “I don't want to get my diagnosis from a computer,” says Goyen. “I want to talk to a real person. If the physician came to the result in this decision based off AI, I love it, it’s great, but I need this human interface. That personal relationship will never go away.”