Interview
How Owkin trained its AI on tissue data without having any tissue or data
French biotech company Owkin is among those at the vanguard of using artificial intelligence (AI) to advance healthcare. Stu Robarts hears more from Andrew Pierce.
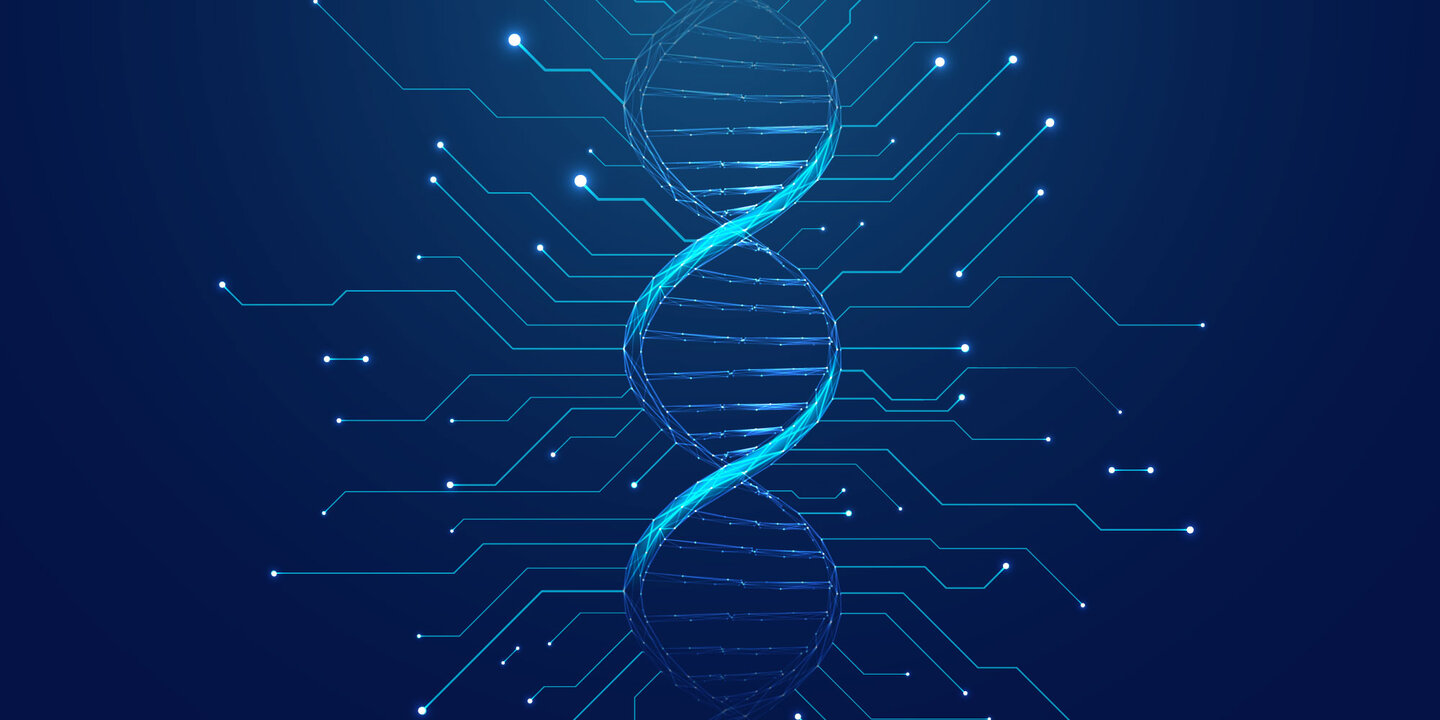
Credit: AntonKhrupinArt / Shutterstock
Describing itself as “the first end-to-end AI biotech”, the firm employs the technology in various ways and at various points of the clinical process. Biomarkers are identified to inform drug discovery, existing drugs are matched to patient subgroups, methodologies are optimised and data harnessed to increase the success of clinical trials and patients are screened to help with diagnostics.
Partnered with the likes of Sanofi, BMS and MSD, as well as over 60 academic institutions globally, Owkin provides a glimpse of a new AI-aided future for healthcare. Andrew Pierce, the company’s SVP for drug discovery and development, tells us about the founding purpose of the business, how it employs AI and his hopes for the future of the technology in healthcare. The interview has been edited for brevity and clarity.
Stu Robarts: What is Owkin’s story?
Andrew Pierce: The company came about as a joint venture between data science and clinical medicine. The idea was to use the emerging field of AI to help improve outcomes in the medicine world, and the initial focus for the business in that regard was oncology.
The idea was also that what is really driving the best applications of AI is when it has access to very high-quality data sets to work with. Owkin recognised that very early in the game and came up with what I thought was a quite clever way to get the underpinning data. We have this large project called Mosaic, which is basically the world's largest dataset for spatially resolved biomarkers in tissues that are of relevance to cancer across a number of different tumour types, and they've been analysed in extraordinary detail. This all provides the foundational data that the AI uses to help shape clinical development.
There's a barrier to assembling high-quality data sets of this nature. Owkin doesn't have any tissue – the tissue is at hospitals all over the world. If you go to a hospital and you say, “Can I have your tissue?” the sort of reflexive answer is, “Well, no, why? Why would we do that? And doesn't that compromise patient privacy and our own intellectual property?”
The way around that was that Owkin has really pioneered this federated learning approach, and the idea for this is that the tissue doesn't need to leave the hospitals, and the data doesn't need to leave the hospitals either. The AI framework comes to the hospital and does what it needs to do to learn enough to help with clinical development, but the data, the actual foundational data, doesn't leave the hospital – just the conclusions from the data leave the hospital. That really was the key to unlocking the willingness of world-leading medical centres to agree to sign up to this. I think at last count there are something like 60 different medical centres all over the world that have contributed the underpinning data that has led to the AI machine that can draw these conclusions.
I think the real difference maker for Owkin is that we have the conclusions from the data and others don't because they're still fighting over the data itself. We found a way to not have to fight over the data and just get to the conclusions, the solutions.
Stu Robarts: What makes AI suited for use in complex biology?
Andrew Pierce: It's not just number-crunching on big data. People have done number crunching on big data for a long time, and that's wonderful, but it's really sort of a game-changing paradigm. It's a different way of coming at the data. One of the usual things that people say about AI is it can give you correct conclusions, but it doesn't explain how it got to those conclusions. In some ways, I think that that's still true, and that's why to someone like me, who's a biologist and a scientist and not an AI data person, it really does look like magic in some of the things that it can do.
Here's an example. Everybody who has cancer will have an image of their cancer at the cellular level. That's how cancer is diagnosed. You take a tissue sample, you do an H&E stain on it – this has been true for like 150 years – and then a pathologist looks at that, and based on the pattern says, “This is cancer,” or “This is not cancer.”
With the pathologist, this is not AI. They've been trained for a long time so they can look at these patterns, and their brain says what the pattern says to them – and that's what the AI does as well. It's a pattern-matching thing, but, because it's machine-driven, it is able to look in much more depth and much greater detail and to consider relationships much more broadly than what a human would do.
By sending the AI thousands and thousands of images of these slides that a pathologist would look at and lining those up with known conclusions, the AI is able to train itself to essentially do the equivalent of helping the pathologist be even better at this. A pathologist will be able to look at a slide from colon cancer and say, “This is colon cancer,” or, “It's not colon cancer.” The AI can do that too, but the AI can also say, “Not only is this colon cancer, but this is colon cancer that has a defect in DNA mismatch repair.” That's something that pathologists can't call, so this is helping the pathologist take it to the next level. Why that matters is because there are drugs that work brilliantly well in colorectal cancer but only in the context of this special pathway being broken, a defect in DNA mismatch repair.
Usually, there are like five different ways to assess the status of the molecular characteristics of this disease. You can do DNA sequencing, you can do immunosochemistry, there are multiple different assays, or you can just take this image that everybody's already got – this H&E slide that people have been doing for 100-plus years. But because the AI has learned what mismatch repair deficient cancer looks like on this type of slide, it's able to make that call. If the AI looks at it and says this is probably this specific molecular subtype that will respond to this drug, then you do confirmatory testing, and then you prescribe the drug. But if the AI looks at it and says I don't think this is this subtype, then that is almost certainly true, and you don't need to do further testing. That's a little example of how the application of AI has really enabled streamlining of determining which specific patients would benefit from which specific types of drugs.
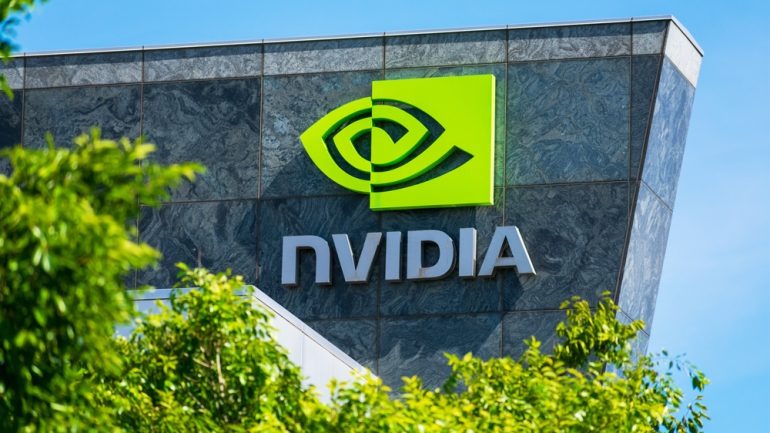
NVIDIA briefly became the most valuable company in the world last month. Credit: Michael Vi / Shutterstock
AI provides tools and increases our capacity to process data. So instead of searching and reading every single paper, you can ask an AI agent to summarise a lot of them. It helps humans make sense of such a vast volume of information and literature. But designing new drugs is a really complex endeavour. AI agents can help in some tasks but are not the only tool.
AI is speeding up the drug development process by simplifying some steps to allow better choices. But it’s not a one-size-fits-all solution.
Stu Robarts: How is Owkin’s description of itself as “the first end-to-end AI biotech” exemplified through its use of AI elsewhere?
Andrew Pierce: What that means is that we have our own drugs. We're running our own clinical studies. The first of those will initiate very shortly. That’s different from a lot of AI companies. It’s one thing to put AI scientists in a room with a bunch of machines, but it's different when you're doing it and then also using it yourself in the clinic.
We're using AI to inform our own decisions in clinical development, in addition to being able to partner with other pharma companies to help them make better AI-informed and enabled decisions in their studies. We're really putting our money, our effort and our new medicines to the test explicitly in this context, and that's sort of what the “end-to-end” means. It's not just the AI, it's the AI and clinical development, and we have hands-on experience with both of those things.
Stu Robarts: What broader impact do you think AI is going to have across the healthcare sector?
Andrew Pierce: I'm super excited by the technology. I think it is going to be transformative. I think you're going to see more drugs, better drugs, and faster drug development. It's going to take a while for that to actually become apparent to the public, because there’s still is a process of drug development, but I'm super optimistic for the future.
Specifically, with Owkin, this is my first foray into AI-enabled drug development, and I'm brought in more for the drug development piece, but I'm super excited about having an integrated approach where the AI informs what we do in the clinic. Then there's this so-called reverse translation approach, where the clinical data and all the biomarker data that we generate that gets fed back to the AI engines to help them be even better, so it creates this virtual cycle. It's traditionally called bench to bedside and back again, and it's really going to turbocharge all of that.
In part, that's why I was super excited to take this opportunity to join Owkin and help them turn this into a reality. And you know, I think others in similar settings are just as excited if you talk to other people involved in this sort of AI-enabled medicine world – particularly, I think, in oncology where the need is so dire and the historical success rates are so low. That's why this has such game-changer potential. So yeah, I'm really optimistic about the future.