AI
IQVIA on AI’s potential to match patients to trials and improve trial diversity
Lucas Glass, vice-president of IQVIA’s analytics center of excellence talks to Kezia Parkins about matching patients to trials with AI and how the technology can improve patient diversity rather than hinder it.
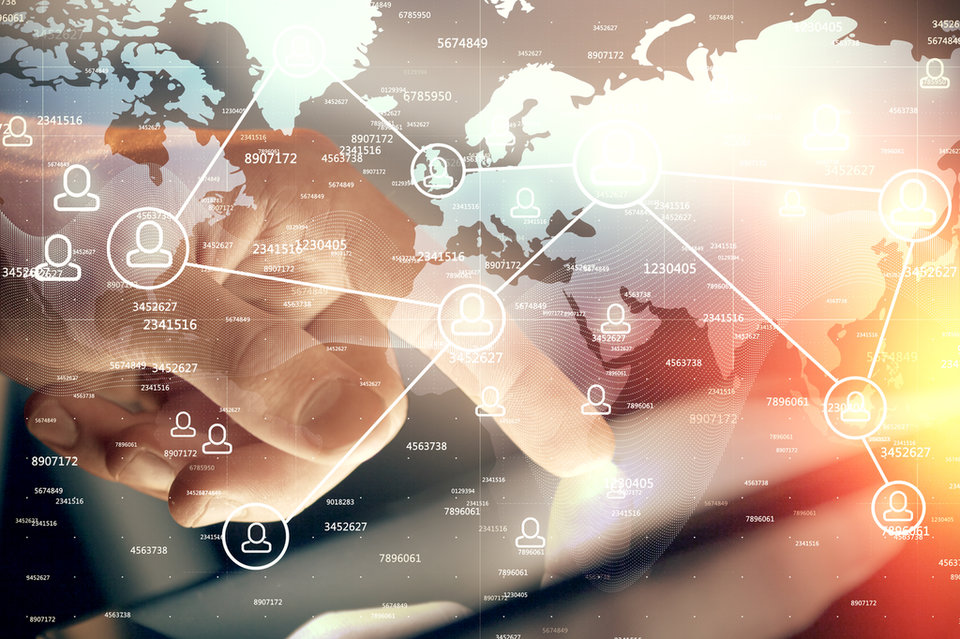
A
rtificial intelligence (AI) is increasingly being harnessed to increase the operational efficiency of clinical trials, accelerate drug discovery and reduce costs. It can be used to unlock information from a diverse range of data sources and support faster and more meaningful patient recruitment.
One of the ways that AI can aid in patient recruitment - one of the most challenging areas of running trials - is with patient matching. By introducing AI into the clinical care setting, doctors could easily be notified of potential trials their patients could benefit from, especially those with unmet needs where there may be few options available on the market.
A company at the cutting edge of this solution is IQVIA. We sat down with the vice-president of the firm’s analytics center of excellence (ACoE), Lucas Glass, to discuss matching patients to trials with AI, the issue of trial diversity and some of the challenges in hiring talent to the AI clinical research space.
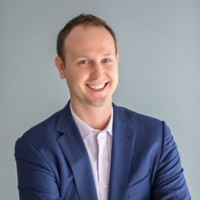
Lucas Glass, vice-president of IQVIA
Kezia Parkins:
How can AI be used to match patients to a clinical trial?
Lucas Glass:
There's really two paradigms that we've seen play out. One is a site that's looking through their patients to find potential participants that match a given trial. ‘I have a trial and I'm looking for patients’ is a very common mechanism. The other paradigm is ‘I'm a patient and I'm looking for a trial’.
Machine learning has been playing pretty aggressively in both those spaces. From a very crude perspective, 'I have a trial and I'm looking for patients' has been much more robust because the funding mechanisms in that space are very clear. But, for patients trying to search for trials, the place that's been built historically is clinicaltrials.gov, which is not particularly patient-friendly.
People continuously try to solve this problem, but it's one thing to find some sort of problem/solution fit, but another to find a product/market fit. I know that we are very focused on it and trying to address it, but it is a continuing ongoing challenge.
How can AI be integrated into the clinical care setting to match patients to a trial?
Where I've seen some of the plays happening is when we can integrate this into the workflow of a doctor by the bedside of a patient. Some sort of alert that says, 'Hey, did you know there are these studies that this patient might benefit from?' Then the doctor who is very familiar with medicines out on the market, but often not with those that aren't yet, can help establish that.
Figuring out some way to insert that into the workflow without being annoying to the doctor is important. The last thing doctors want is more pop-ups and technology.
I imagine it as a bit more of a passive thing. The algorithms aren't the problem. It's that clinical workflow, that's the real challenge.
Where would you say we are with the adoption curve of AI in the industry when it comes to matching patients to clinical trials?
As an industry, we're starting to see a fair amount of investment coming into the space whether it be in big pharma, or even in the venture capital world. There's going to be some breakthroughs in the next two, three years that have a big impact because I see a lot of work and effort going into it.
No one's really cracked through and found the stride of lots of patients signing up and finding the right trials, but we are close. One of the reasons I'm at IQVIA is that I think we will be the ones that break through that threshold.
Patient diversity is something that is being spoken about a lot more in the industry. And then something else that we also talk about a lot is how AI can be quite racist or biased.
I would say AI is non-moral.
So it's the people behind the program?
Yes, it [AI] mimics previous behaviours - bias behaviours. AI is not very intelligent, don't tell anybody.
In that case, how can we ensure that the use of AI helps patient diversity rather than hinders it?
This is a big research area for me now - diversity in clinical trials. There's some really good work coming out of the University of Illinois and Cornell around fairness in AI.
It's not specific to diversity. It's just the concept of constraining the AI to make sure that it's focused on reaching some sort of distribution. It could be completely arbitrary making sure you're meeting a distribution of apples versus oranges. But the applications in the diversity of clinical trials is really beneficial.
For us, some of the spaces that we're focused on - and this is not trial matching specifically, because if I'm helping patients find a trial, I would help all patients find trials regardless of ethnicity - is figuring out which doctors I would pull into my study. I would make sure that my AI is paying attention to the diversity of the doctors’ patient panels. I don't want to go after physicians that are only treating the white patient population. I want to get physicians that are treating a diverse patient population.
And some of these fairness in AI methodologies from these schools and research groups that I mentioned are really doing a great job at that. We've been working to incorporate that into some of our own AI for site selection and patient recruitment.
The industry has been talking about the silos within data and not having access to data in other countries for some time. Is that something that IQVIA struggles with? Or as a leader in this space, do you have enough access to data that your algorithms are trained well?
I work in R&D and we have a lot of use cases that benefit from all of these real-world global data assets. However, R&D doesn't historically have the experience working with assets and all the privacy and technology restrictions. It's a very complicated system to navigate.
But, when I created the ACoE with my leadership, we had this huge luxury - we've got this global organisation, and half of our company is focused on country-specific data, data products, data solutions, and so I literally hired three people in every country to help build the solutions and ensure we're completely compliant with the local regulations because every country's data and regulatory landscape is completely different.
We placed the big bet that that's going to be beneficial and we feel it really has been in incorporating all these global data assets into how we run our business and our clinical trials.
That's probably the biggest differentiation that IQVIA has, is those really rich, diverse set of countries and expertise in those countries that I can bring to bear for my R&D use cases. If I'm trying to extend it to a specific country, I have somebody I call up and say, ‘Hey, can I borrow somebody from your organisation to help me expand into your country?’
Is hiring the right people a big challenge in this space?
Yes. Not just on the front of knowing global data assets, which is super complicated - some data have different languages and different data assets - but then there are the complex problems of machine learning. Machine learning is hard!
There are lots of really bright students graduating every year from lots of universities in the space. But getting students and graduates in the AI space, and then even more difficult, well established, experienced AI professionals who know clinical trials, is like finding a needle in a haystack.
A lot of my talent gets poached by pharma companies. I'm very happy for my team members to get big, exciting opportunities, but because there's just not a lot of talent that has both that AI background, as well as the clinical domain expertise - we spend a lot of time hiring AI professionals and then training them about clinical trials.